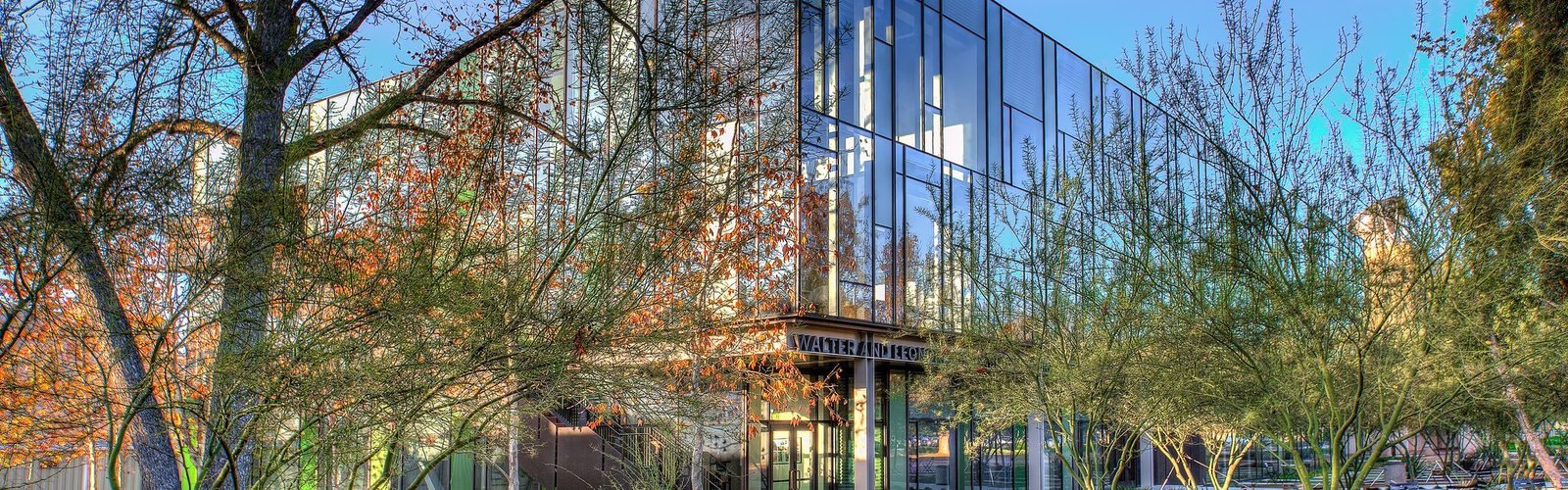
Applied Math Reunion 2023
in Applied Mathematics and its Applications
November 11-12, 2023
Caltech -- Pasadena, California
With the rapid developments in technology, applied and computational mathematics has played an important and unprecedented role in many scientific disciplines. The main objective of this workshop is to bring together researchers, students, and practitioners with interest in the theoretical, computational, and practical aspects in applied and computational mathematics. Another purpose is to have a reunion of the alumni of Applied and Computational Mathematics at Caltech. This workshop will cover recent progress, stimulate new ideas, and facilitate interdisciplinary collaborations. It will emphasize the crucial and unique role of mathematical insights in advanced algorithm design and novel real-world applications.
This workshop will be held at Caltech on November 11-12, 2023 (Saturday-Sunday). The meeting will feature distinguished lectures from leaders in the related fields, and panel discussions on future directions.
Registration
Registration for the Workshop is now closed. If you have any questions, please contact Diana Bohler.
Program
The location for all talks will be Annenberg 105 (Auditorium).
Click on the arrows in the schedule below to toggle for more information.
Saturday, November 11
Morning Session Chair: Tom Hou, Caltech
8:00-8:45am
--
8:45-9:00am
--
9:00-9:30am
Towards Seamless Numerical Homogenization
The computational challenge from multiscale differential equations has inspired development of a variety of methodologies. Many of them require scale separation but there are also efforts to reduce the dependence on substantial scale gaps. One such class of techniques is the, so called, seamless methods for multiscale dynamical systems. We will prove equivalence between such dynamical systems and one dimensional elliptic multiscale problems. A simple multidimensional generalization leads to a new class of numerical methods for homogenization type problems. This has some similarity to turbulence modeling and the Car-Parinello technique for molecular dynamics. We will give error estimates and simple numerical examples.
9:30-9:50am
Multimodal Sampling via Approximate Symmetries
Sampling from multimodal distributions is a challenging task in scientific computing. When a distribution has an exact symmetry between the modes, direct jumps among them can accelerate the samplings significantly. However, the distributions from most applications do not have exact symmetries. This paper considers the distributions with approximate symmetries. We first construct an exactly symmetric reference distribution from the target one by averaging over the group orbit associated with the approximate symmetry. Next, we can apply the multilevel Monte Carlo methods by constructing a continuation path between the reference and target distributions. We discuss how to implement these steps with annealed importance sampling and tempered transitions. Compared with traditional multilevel methods, the proposed approach can be more effective since the reference and target distributions are much closer.
9:50-10:10am
Multicontinuum homogenization
I will give some overview of our research on multicontinuum homogenization that arise in multiscale problems. The main idea is to construct multiple multiscale basis functions. I will discuss both scale separation and no-scale separation cases.
10:10-10:40am
--
10:40-11:10am
[Click here to view slides]
In-Context Operator Networks: Towards Large Scientific Learning Models Scientific Learn
Joint work with Liu Yang, Siting Liu and Tinwei Meng.
11:10-11:30am
Multi-Operator Learning and Expression Generation
Approximating nonlinear ordinary differential equations using a neural network provides an efficient tool for certain scientific computing tasks, including real-time predictions, inverse problems, and surrogate modeling. The focus thus far has been on embedding a single solution operator, associated to one (possibly parametric) differential equation, into a neural network. However, it is often the case that families of differential equations share similar behavior and thus we can leverage this to train one neural network to represent multiple distinct tasks. In this talk, we will discuss how to learn maps from multimodal inputs to multimodal outputs that are capable of generating both numerical predictions of dynamical systems and mathematical equations over multiple distinct differential equations.
11:30-11:50am
Recent development on singularity formation in incompressible fluids
I will talk about recent development on singularity formation in incompressible fluids.
11:50am-12:10pm
[Click here to view slides]
Matrix decompositions in DNA sequence analysis
In the past 15 years, astounding advances have been made in the DNA sequencing technology. This has enabled large-scale sequencing of human genomes, generating terabyte-scale datasets. I will describe one approach for interpreting DNA mutations in cancer patients involving non-negative matrix factorization.
12:10-2:00pm
Please see below for a list of suggested dining establishments both on- and off-campus.
Afternoon Chair: Houman Owhadi, Caltech
2:00-2:30pm
The Historical Development and Transformation of Applied and Computational Mathematics at Caltech
In this talk, I will give a brief review on the history and transformation of Applied and Computational Mathematics at Caltech. The Applied Math Option (AMa) was established in 1967 by Gerald Whitham and a few founding faculty members, Philip Saffman, Donald Cohen, Herbert Keller, and Joel Franklin. Heinz-Otto Kreiss joined AMa from 1978 to 1987 and Dan Meiron joined in 1985. Since 1992, there has been a new wave of hiring, including Tom Hou (1993), Oscar Bruno (1995), Niles Pierce (1999), Emmanuel Candes (2000), Houman Owhadi (2003), Joel Tropp (2006), Venkat Chandrasekaran (2012), Andrew Stuart (2016), and Franca Hoffmann (2022). The Applied Mathematics Option was renamed to "Applied and Computational Mathematics" (ACM) in 2001, and ACM was merged with CS and CDS to form the Department of Computing and Mathematical Sciences (CMS) in 2010 with a strong focus on the mathematics of information and data science. I will highlight the accomplishments of some of our faculty members, our former Ph.D. students and postdocs, including those from my research group.
2:30-3:00pm
The relation between ranked and unranked eigenportfolios
We will show how portfolios with unranked equities returns data and the corresponding ones ranked by capitalization are related. We will show that this theoretical, model free, analysis is is in fact quite consistent with portfolios of actual US equities data. Applications will be discussed.
3:00-3:20pm
Recovery phenomena with symmetric autoencoders
Is it possible to guess what a scene in an image would look like if the picture was taken from a different angle? Would it sound like you if an AI generated a deepfake of your voice? Can we find the solution of a PDE we have never seen, if we collect enough solutions of nearby equations? These questions seem to fit in a common mathematical framework of estimation of low-dimensional latent processes under maps of controlled complexity. After reviewing known results in the context of generative priors, I will explain how to formulate recovery guarantees for symmetric autoencoders using tools from applied probability and approximation theory. Joint work with Borjan Geshkovski (MIT).
3:20-3:40pm
A group photo will be taken of the Workshop attendees.
3:40-4:00pm
Inverse wave scattering via reduced order modeling
I will describe briefly a novel approach to inverse wave scattering, which uses an array of sensors to probe an unknown heterogeneous medium with signals and measures the scattered waves. The approach uses tools from data driven reduced order modeling to estimate the wave field at points inside the inaccessible medium that we wish to determine.
I will show how we can use this wave to obtain a better formulation of inverse wave scattering than the typical nonlinear least squares data fitting. The performance of the method is illustrated with a few known challenging examples.
4:00-4:20pm
Consensus Based Optimization and Sampling
Particle methods provide a powerful paradigm for solving complex global optimization problems leading to highly parallelizable algorithms. Despite widespread and growing adoption, theory underpinning their behavior has been mainly based on meta-heuristics. In application settings involving black-box procedures, or where gradients are too costly to obtain, one relies on derivative-free approaches instead. This talk will focus on two recent techniques, consensus-based optimization and consensus-based sampling. We explain how these methods can be used for the following two goals: (i) generating approximate samples from a given target distribution, and (ii) optimizing a given objective function. They circumvent the need for gradients via Laplace's principle. We investigate the properties of this family of methods in terms of various parameter choices and present an overview of recent advances in the field.
4:20-4:30pm
--
4:30-6:00pm
Chair: Tony Chan, KAUST
Panelists:
Franca Hoffmann
Gang Hu
Zhenzhen Li
Pengfei Liu
Gaby Stredie
Xiao-Hui Wu
Mike Yan
Pengchuan Zhang
6:30-9:30pm
Attendees can share their stories of Caltech along with their own life stories after Caltech.
Registration for the Banquet has closed. Registered Banquet attendees will receive a message containing Banquet logistics shortly before Saturday, November 11th.
Please contact Diana Bohler with any questions.
Sunday, November 12, 2023
Morning Chair -- First Session: Tony Chan, KAUST
9:00-9:30am
[Click here to view slides]
Optimization of the Boltzmann Equation
The kinetics of rarefied gases and plasmas are described by the Boltzmann equation and numerically approximated by the Direct Simulation Monte Carlo (DSMC) method. We present an optimization method for DSMC, derived from an augmented Lagrangian. After a forward (in time) solution of DSMC, adjoint variables are found by a backwards solver. They are equal to velocity derivatives of an objective function, which can then be optimized. This is joint work with Yunan Yang (Cornell) and Denis Silantyev (U Colorado, Colorado Springs).
9:30-9:50am
Some Problems in General Relativity and Memories of Caltech during 1993-2005
This lecture will focus on some research problems in mathematical and numerical general relativity (GR) I was first exposed to as a postdoc at Caltech in (at the time) Applied Mathematics. I was mentored primarily by Herb Keller, and his first instructions were to attend Kip Thorne's relativity class (Physics 236) that Fall (1993). I will outline a number of interesting problems in numerical analysis that came out of my interactions with Herb, Kip, and many applied math faculty over the four year period 1993-1997, and formed the basis for many of my research projects during my time at UC Irvine and UC San Diego (1997 through today). An additional 2-year leave at Caltech during 2003-2005 led to a new direction involving some open mathematical problems in GR, leading to other projects that have formed a second center of mass in my research program since 2008. The format of my lecture will be a little unusual, with my technical presentation intertwined with fond memories of interactions with mentors, collaborators, and friends at Caltech during the period 1993-2005.
9:50-10:10am
Optimal Transport Maps for Conditional Simulation
Optimal transport (OT) considers the problem of finding a mapping that warps a reference probability measure to a target of interest. Such a map can naturally be viewed as a generative model in the context of machine learning applications making the framework of OT a natural candidate for the analysis of generative modeling. In this talk I will discuss the foundational theory of a particular class of OT problems where the resulting map does not only transport the reference measure to the target but is also capable of providing samples from certain conditionals of the target. This problem is very interesting in the context of Bayesian inference and in particular in the setting of amortized and simulation based inference.
10:10-10:40am
--
Morning Chair -- Second Session: Tom Hou, Caltech
10:40-11:10am
Runge-Kutta Methods are Stable
We discuss the stability of Runge-Kutta methods for large systems of ODEs.
11:10-11:30am
Speeding up gradient flows on probability measure space
In the past decade, there has been a significant shift in the types of mathematical objects under investigation, moving from vectors and matrices in Euclidean space to functions residing in Hilbert or Banach spaces, and ultimately extending to probability measures within the probability measure space. Many questions that were originally posed in the context of linear function spaces are now being revisited in the realm of probability measures. One such question is to the efficiently find a probability measure that minimizes a given objective functional.
In Euclidean space, we devised optimization techniques like gradient descent and introduced momentum-based methods to accelerate convergence. Now, the question arises: Can we employ analogous strategies to expedite convergence within the probability measure space?
In this presentation, we provide an affirmative answer to this question. Specifically, we present a series of momentum-inspired acceleration method under the framework of Hamiltonian flow, and we prove the new class of method can achieve arbitrary high-order of convergence. This opens the door of developing methods beyond standard gradient flow.
11:30-11:50am
[Click here to view slides]
Numerical methods for geometric motion
We consider the evolution of curves in 2D. We describe it as geometric motion if the evolution only depends on the shape of the curve. There are applications in material science (the evolution of microstructure in materials), biochemistry, and image processing. Two new gradient flow models are derived and their numerical implementation in a general computational framework is described.
11:50am-12:10pm
Machine Learning-enabled Self-Consistent Field Theory for Soft Materials
Self Consistency Field Theory (SCFT) has been a powerful tool to study soft materials like polymers. However, SCFT simulations are a complex and computationally costly process. Exploring the vast design space of polymers via SCFT is often impractical. We will discuss in this talk our recent efforts to leverage SCFT with Machine Learning to accelerate many important downstream tasks, such as the discovery of new phases.
12:10-2:00pm
Please see below for a list of suggested dining establishments both on- and off-campus.
Afternoon Chair: Haomin Zhou, Georgia Tech
2:00-2:20pm
[Click here to view slides]
Parameterized Wasserstein Geometric Flow
In this presentation, I will give a brief introduction to a new parameterization strategy that can be used to design algorithms simulating geometric flows on Wasserstein manifold, the probability density space equipped with optimal transport metric. The framework leverages the theory of optimal transport and the techniques like the push-forward operators and neural networks, leading to a system of ODEs for the parameters of neural networks. Theoretical error bounds measured in Wasserstein metric are provided. The resulting methods are mesh-less, basis-less, sample-based schemes that scale well to higher dimensional problems. We demonstrate their performance in Wasserstein gradient flows such as Fokker-Planck equation, and Wasserstein Hamiltonian flow like Schrodinger equations.
2:20-2:40pm
[Click here to view slides]
A Nontraditional Regularity Criteria for the 3D Navier-Stokes Equations
We present a nontraditional regularity criterion for the global well-posedness problem of the three dimensional Navier-Stokes equations in the whole space. The main novelty of this new criterion is that it involves the shape of the magnitude of the velocity. More specifically, we prove that if for every fixed time in (0,T), the region of high velocity, appropriately defined with a parameter q, shrinks fast enough as q↗∞, then the solution stays regular beyond T.
This is joint work with Prof. Chuong V. Tran of the University of St. Andrews, United Kingdom.
2:40-3:00pm
[Click here to view slides]
Stochasticity of Deterministic Gradient Descent: Quantitative Local Min Escape in Multiscale Landscape
This talk will discuss one of the many nontrivial but often pleasant effects of large learning rates, which are commonly used in machine learning practice for improved empirical performances but defy traditional theoretical analyses. More specifically, I will quantify how large learning rates can help gradient descent escape local minima, via chaotic dynamics, which provides an alternative to the commonly known escape mechanism due to noises from stochastic gradients.
3:00-3:20pm
[Click here to view slides]
Information geometric regularization of the barotropic Euler equation
This talk presents an inviscid regularization for mitigating shock formation in the multidimensional barotropic Euler equations based on ideas from geometric hydrodynamics, interior point methods for semidefinite programming, and the information geometry of Amari and Chentsov. In Lagrangian coordinates, the solutions of Euler's equations are paths on the manifold of diffeomorphisms. Shocks form when the deformation map reaches the boundary of this manifold. Shock formation thus arises from the geodesic incompleteness of the latter. In this work, we regularize the barotropic Euler equation by modifying the geometry of the diffeomorphism manifold. In the modified geometry, geodesics do not cross the boundary of the manifold but instead approximate it asymptotically. This modified geometry is motivated by the log-determinant barrier function in semidefinite programming and the information geometry of the fluid density. By re-expressing the resulting equation in Eulerian coordinates we obtain an information geometric regularization of the original conservation law. We provide numerical evidence that this regularization prevents shock formation while preserving the long-term behavior of the solutions.
3:20-3:40pm
--
3:40-4:00pm
[Click here to view slides]
Generalized multiscale finite element method for a class of nonlinear flow equations
In this talk we present a Constraint Energy Minimization Generalized Multiscale Finite Element Method (CEM-GMsFEM) for solving single-phase non-linear compressible flows in highly heterogeneous media. The construction of CEM-GMsFEM hinges on two crucial steps: First, the auxiliary space is constructed by solving local spectral problems, where the basis functions corresponding to small eigenvalues are captured. Then the basis functions are obtained by solving local energy minimization problems over the oversampling domains using the auxiliary space. The basis functions have exponential decay outside the corresponding local oversampling regions. The convergence of the proposed method is provided, and we show that this convergence only depends on the coarse grid size and is independent of the heterogeneities. The research is partially supported by the Hong Kong RGC General Research Fund (Projects: 14305222 and 14304021).
4:00-4:20pm
Dynamics of fluid's cohomology
We present a topological analysis of the vorticity formulation of the incompressible Euler equation. In particular, we elucidate the equations of motion for the often-omitted cohomology component of the velocity on non-simply-connected domains. These equations have nontrivial coupling with the vorticity, which is crucial for characterizing correct vortex motions with presence of tunnels or islands in the domain. The dynamics of fluid's cohomology reveals the curvature of the commutator subgroup of the Lie group of volume-preserving diffeomorphisms, and it is also associated with new conservation laws as Casimir invariants. Additional results include new analytical solutions of the Euler equations that are related to the Hilbert transform; and the first general vortex-streamfunction-based numerical method on curved surfaces with arbitrary genus and boundaries that is consistent with the Euler equation.
4:20-4:40pm
[Click here to view slides]
Efficient Interacting Particle Methods for Computing Near Singular Solutions of Keller-Segel Chemotaxis Systems and High-Dimensional Eigenvalue Problems
Mesh-based methods, such as the finite element method and spectral methods, often encounter significant challenges when solving PDEs with near singular solutions or in high-dimensional spaces. This talk presents an efficient interacting particle method inspired by our recent developments in particle-based techniques for computing effective diffusivities in chaotic flows and KPP front speeds of reaction-diffusion-advection equations. The method is applied to compute aggregation patterns and near singular solutions of the Keller-Segel (KS) chemotaxis system in three-dimensional space, considering both the parabolic-elliptic and parabolic-parabolic types of KS systems. Additionally, the interacting particle method is employed to calculate the principal eigenvalues of high-dimensional elliptic operators, enabling the analysis of the large-time growth rate of the entropy functional, which quantifies the time reversal of SDEs in high dimensions under vanishing noise. Numerical experiments are presented to demonstrate the performance of the proposed methods. Furthermore, this talk introduces the DeepParticle method, a deep learning method for learning and generating the distributions of solutions under variations of physical parameters.
4:40-5:00pm
Equivalent Extensions of Partial Differential Equations on Curves
or Surfaces
In this talk, we propose a methodology that extends the energy
function defined on surfaces to the energy function defined on the
nearby tubular neighborhood that gives the same energy when inputting
the constant-along-normal extension. Furthermore, the extended energy
function gives the same minimizer as which the original energy function
gives in the sense of restriction on the surface. This new approach
connects the original energy function to an extended energy function and
provides a good framework to solve PEDs numerically on Cartesian grids.
Recently, we have used the sign distance function defined in a
narrowband near the moving interface to represent the evolution of the
curve. We derive the equivalent evolution equations of the distance
function in the narrowband. The novelty of the work is to determine the
equivalent evolution equation on Cartesian girds without extra
conditions or constraints. The proposed method extends the differential
operators appropriately so that the solutions on the narrowband are the
distance function of the solution to the original mean flow solution.
Furthermore, the extended solution carries the correct geometric
information, such as distance and curvature, on Cartesian grids. Some
experiments confirm that the proposed method is convergent numerically.
This is a joint work with Richard Tsai, Ming-Chih Lai, Shih-Hsuan Hsu,
Chun-Chieh Lin.
End of the Workshop.
Organizing Committee
Thomas Hou, Chair | Caltech |
---|---|
Houman Owhadi | Caltech |
Peter Schröder | Caltech |
Andrew Stuart | Caltech |
Haomin Zhou | Georgia Tech |
Local Accommodations, Directions, and Parking
Hotel Dena
303 Cordova St, Pasadena, CA 91101
Phone: +1 626-469-8100
Hotel Dena will be the location of the Workshop Banquet.
A block of discounted rooms ($182/night) has been reserved at the Hotel Dena for booking by Workshop attendees. To take advantage of this discount, please reserve directly with the hotel via the online discounted workshop booking link.
The Athenaeum
Caltech's on-campus faculty club offers a limited number of hotel rooms, which may be more expensive than other local options. Reservations at the Athenaeum must be arranged by the Workshop organizers directly. If you are interested in reserving a room at the Athenaeum, please contact us.
The Saga Motor Hotel -- ~0.6 miles from Workshop Venue at Caltech
1633 E Colorado Blvd., Pasadena, CA 91106
www.thesagamotorhotel.com
(626) 795-0431
Hyatt Place Pasadena -- ~1.4 miles from Venue
399 E Green St, Pasadena, CA 91101
www.hyatt.com
(626) 788-9108
Sheraton Pasadena -- ~1.5 miles from Venue
303 Cordova St, Pasadena, CA 91101
www.marriott.com
(626) 469-8100
Westin Pasadena -- ~1.9 miles from Venue
191 N Los Robles Ave, Pasadena, CA 91101
www.marriott.com
(626) 792-2727
Courtyard by Marriott Pasadena/Old Town -- ~2.7 miles from Venue
180 N Fair Oaks Ave, Pasadena, CA 91103
www.marriott.com
(626) 403-7600
The Workshop talks will be held in Room 105 of the Annenberg Center for Information Science and Technology, building #16 on a campus map.
Please refer to the Center's location on Google maps for directions and navigation.
Visitors traveling from LAX (Los Angeles International Airport) and BUR (Hollywood Burbank Airport) to Caltech tend to choose a ride service like Uber or Lyft. Alternatively, you can use SuperShuttle (advance reservation recommended via supershuttle.com), rent a car at the airport, or pick up a taxi cab at a designated location at the airport.
- Information about taxi pickup locations can be found below, along with other ground transportation details:
The nearest Caltech parking structure to the Annenberg Center for Information Science and Technology is Structure #4, located at 370 South Holliston Avenue, Pasadena. Parking permits must be displayed in order to park in visitor (unmarked) parking spaces. Permits can be purchased at pay stations located in campus parking lots and structures.
More information about visitor parking can be found at: https://parking.caltech.edu/parking-info/visitor-parking
Lunch during the Workshop will be on your own (not provided by the organizers). Please see below for dining suggestions both on- and off-campus:
On Campus
To view the different dining options on campus along with current hours, please see:
https://dining.caltech.edu/where-to-eat-
Off Campus
There are many great restaurants, bars, and lounges within walking distance of campus, including the Old Town Pasadena vicinity as well as the South Lake Avenue Shopping District.
- South Lake Avenue Shopping District:
- http://www.southlakeavenue.org/business-directory/food-dining/
- South Lake Avenue is within walking distance of campus (approximately 14 minutes), and has many dining options from fast food (Chipotle, Veggie Grill, Panda Express) to more formal full-service establishments.
- For directions to the South Lake Avenue Shopping District vicinity from Caltech's campus, click here.
- Ginger Corner Market: http://gingercornermarket.com/
- Ginger Corner Market is a small café within walking distance of campus (approximately 6 minutes).
- For directions to the Ginger Corner Market from Caltech's campus, please click here.
- Old Town Pasadena: https://www.oldpasadena.org/visit/directory/dine/
- Old Town Pasadena is an approximate 9-minute drive from Caltech's campus.
- For directions to the Old Town Pasadena vicinity from Caltech's campus, please click here.